
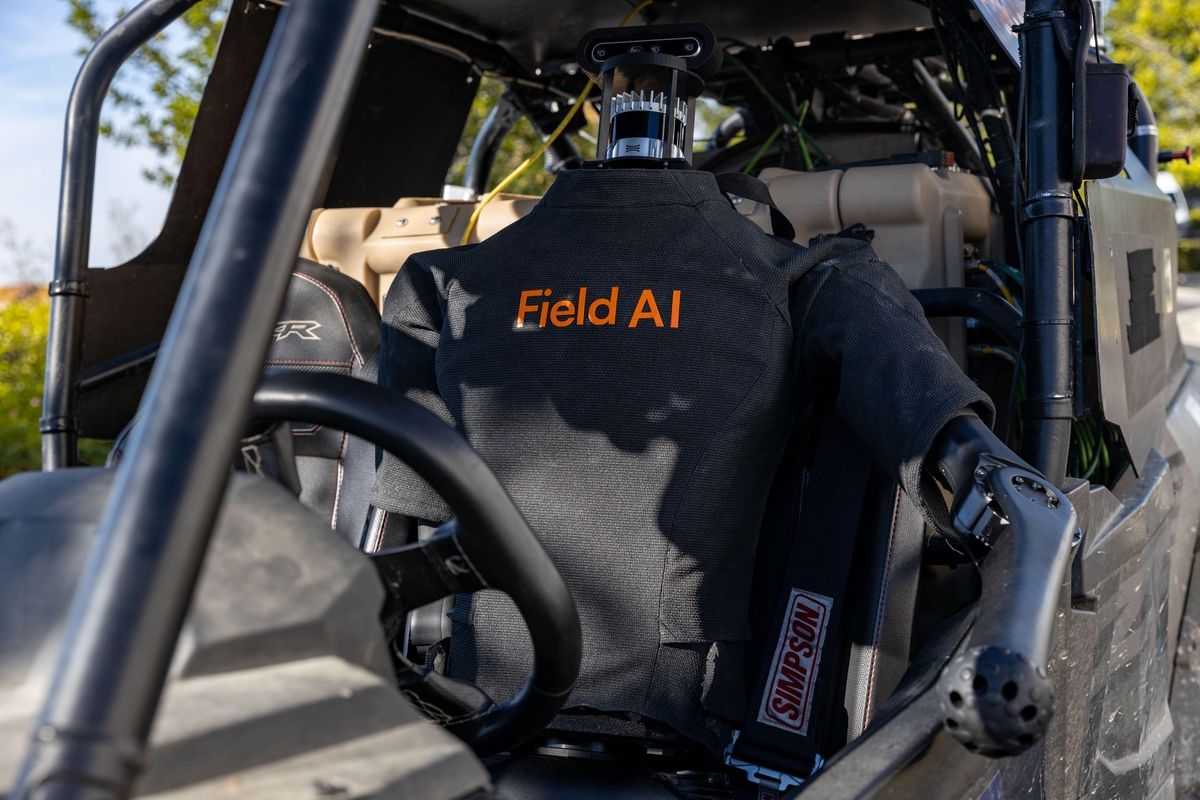
One of the biggest challenges for robotics right now is practical autonomous operation in unstructured environments. That is, doing useful stuff in places your robot hasn’t been before and where things may not be as familiar as your robot might like. Robots thrive on predictability, which has put some irksome restrictions on where and how they can be successfully deployed.
But over the last few years, this has started to change, thanks in large part to a couple of pivotal robotics challenges put on by DARPA. The DARPA Subterranean Challenge ran from 2018 to 2021, putting mobile robots through a series of unstructured underground environments. And the currently ongoing DARPA RACER program tasks autonomous vehicles with navigating long distances off-road. Some extremely impressive technology has been developed through these programs, but there’s always a gap between this cutting-edge research and any real-world applications.
Now, a bunch of the folks involved in these challenges, including experienced roboticists from NASA, DARPA, Google DeepMind, Amazon, and Cruise (to name just a few places) are applying everything that they’ve learned to enable real-world practical autonomy for mobile robots at a startup called Field AI.
Field AI was co-founded by Ali Agha, who previously was the leader of NASA JPL’s Aerial Mobility Group. While at JPL, Agha led Team CoSTAR, which won the DARPA Subterranean Challenge Urban Circuit. Agha has also been the principal investigator for DARPA RACER, first with JPL, and now continuing with Field AI. “Field AI is not just a startup,” Agha tells us. “It’s a culmination of decades of experience in AI and its deployment in the field.”
Unstructured environments are where things are constantly changing, which can play havoc with robots that rely on static maps.
The “field” part in Field AI is what makes Agha’s startup unique. Robots running Field AI’s software are able to handle unstructured, unmapped environments without reliance on prior models, GPS, or human intervention. Obviously, this kind of capability was (and is) of interest to NASA and JPL, which send robots to places where there are no maps, GPS doesn’t exist, and direct human intervention is impossible.
But DARPA SubT demonstrated that similar environments can be found on Earth, too. For instance, mines, natural caves, and the urban underground are all extremely challenging for robots (and even for humans) to navigate. And those are just the most extreme examples: robots that need to operate inside buildings or out in the wilderness have similar challenges understanding where they are, where they’re going, and how to navigate the environment around them.
An autonomous vehicle drives across kilometers of desert with no prior map, no GPS, and no road.Field AI
Despite the difficulty that robots have operating in the field, this is an enormous opportunity that Field AI hopes to address. Robots have already proven their worth in inspection contexts, typically where you either need to make sure that nothing is going wrong across a large industrial site, or for tracking construction progress inside a partially completed building. There’s a lot of value here because the consequences of something getting messed up are expensive or dangerous or both, but the tasks are repetitive and sometimes risky and generally don’t require all that much human insight or creativity.
Uncharted territory as home base
Where Field AI differs from other robotics companies offering these services, as Agha explains, is that his company wants to do these tasks without first having a map that tells the robot where to go. In other words, there’s no lengthy set-up process, and no human supervision, and the robot can adapt to changing and new environments. Really, this is what full autonomy is all about: going anywhere, anytime, without human interaction. “Our customers don’t need to train anything,” Agha says, laying out the company’s vision. “They don’t need to have precise maps. They press a single button, and the robot just discovers every corner of the environment.” This capability is where the DARPA SubT heritage comes in. During the competition, DARPA basically said, ‘here’s the door into the course, we’re not going to tell you anything about what’s back there or even how big it is, just go explore the whole thing and bring us back the info we’ve asked for.’ Agha’s Team CoSTAR did exactly that during the competition, and Field AI is commercializing this capability.
“With our robots, our aim is for you to just deploy it, with no training time needed. And then we can just leave the robots.” —Ali Agha, Field AI
The other tricky thing about these unstructured environments, especially construction environments, is that things are constantly changing, which can play havoc with robots that rely on static maps. “We’re one of the few, if not the only companies that can leave robots for days on continuously changing construction sites with minimal supervision,” Agha tells us. “These sites are very complex—every day there are new items, new challenges, and unexpected events. Construction materials on the ground, scaffolds, forklifts and heavy machinery moving all over the place, nothing you can predict.”
Field AI
Field AI’s approach to this problem is to emphasize environmental understanding over mapping. Agha says that essentially, Field AI is working towards creating “field foundation models” (FFMs) of the physical world, using sensor data as an input. You can think of FFMs as being similar to the foundation models of language, music, and art that other AI companies have created over the past several years, where ingesting a large amount of data from the Internet enables some level of functionality in a domain without requiring specific training for each new situation. Consequently, Field AI’s robots can understand how to move in the world, rather than just where to move. “We look at AI quite differently from what’s mainstream,” Agha explains. “We do very heavy probabilistic modeling.” Much more technical detail would get into Field AI’s IP, says Agha, but the point is that real-time world modeling becomes a byproduct of Field AI’s robots operating in the world rather than a prerequisite for that operation. This makes the robots fast, efficient, and resilient.
Developing field foundation models that robots can use to reliably go almost anywhere requires a lot of real world data, which Field AI has been collecting at industrial and construction sites around the world for the past year. To be clear, they’re collecting the data as part of their commercial operations—these are paying customers that Field AI has already. “In these job sites, it can traditionally take weeks to go around a site and map where every single target of interest that you need to inspect is,” explains Agha. “But with our robots, our aim is for you to just deploy it, with no training time needed. And then we can just leave the robots. This level of autonomy really unlocks a lot of use cases that our customers weren’t even considering, because they thought it was years away.” And the use cases aren’t just about construction or inspection or other areas where we’re already seeing autonomous robotic systems, Agha says. “These technologies hold immense potential.”
There’s obviously demand for this level of autonomy, but Agha says that the other piece of the puzzle that will enable Field AI to leverage a trillion dollar market is the fact that they can do what they do with virtually any platform. Fundamentally, Field AI is a software company—they make sensor payloads that integrate with their autonomy software, but even those payloads are adjustable, ranging from something appropriate for an autonomous vehicle to something that a drone can handle.
Heck, if you decide that you need an autonomous humanoid for some weird reason, Field AI can do that too. While the versatility here is important, according to Agha, what’s even more important is that it means you can focus on platforms that are more affordable, and still expect the same level of autonomous performance, within the constraints of each robot’s design, of course. With control over the full software stack, integrating mobility with high-level planning, decision-making, and mission execution, Agha says that the potential to take advantage of relatively inexpensive robots is what’s going to make the biggest difference towards Field AI’s commercial success.
Same brain, lots of different robots: the Field AI team’s foundation models can be used on robots big, small, expensive, and somewhat less expensive.Field AI
Field AI is already expanding its capabilities, building on some of its recent experience with DARPA RACER by working on deploying robots to inspect pipelines for tens of kilometers and to transport materials across solar farms. With revenue coming in and a substantial chunk of funding, Field AI has even attracted interest from Bill Gates. Field AI’s participation in RACER is ongoing, under a sort of subsidiary company for federal projects called Offroad Autonomy, and in the meantime the commercial side of is targeting expansion to “hundreds” of sites on every platform they can think of, including humanoids.
Reference: https://ift.tt/XwKOa8T
No comments:
Post a Comment